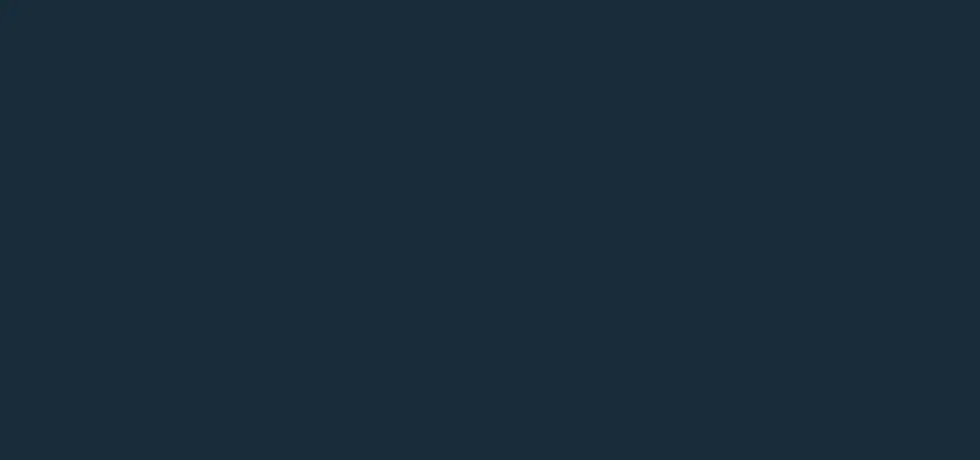.webp)
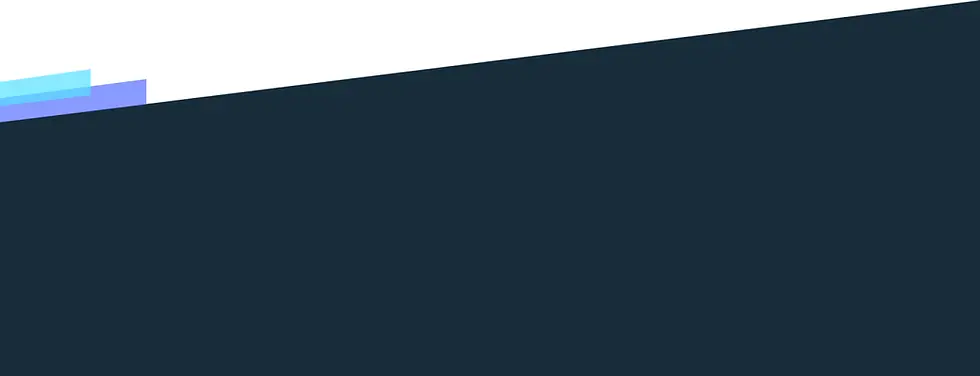.webp)
Challenges Addressed
Inadequate Monitoring: Traditional methods like manual counting and static cameras failed to capture comprehensive data on pedestrian activity, leading to gaps in observation during peak times and major events.
Safety Concerns: The inability to detect risky behaviors, such as jaywalking or overcrowding, in real-time posed a significant risk to public safety. This lack of timely insights contributed to a higher accident rate in critical areas.
Data Overload: While surveillance systems generated vast amounts of video footage, analysts struggled to extract actionable insights efficiently, delaying responses to safety issues.
Inefficient Strategic Planning: The transportation authority’s reliance on manual review processes limited their ability to make data-driven decisions for long-term safety improvements and infrastructure planning.
NEXA's Approach
NEXA developed a machine learning-based motion detection system tailored to the needs of the city transportation authority. This solution utilized advanced computer vision algorithms to process real-time video feeds, enabling accurate and dynamic analysis of pedestrian movements. The system identified unusual behaviors, such as overcrowding or irregular patterns, and provided instant alerts to mitigate potential risks. Additionally, the system generated detailed analytics and reports, offering data-driven insights to improve traffic flow and pedestrian safety.

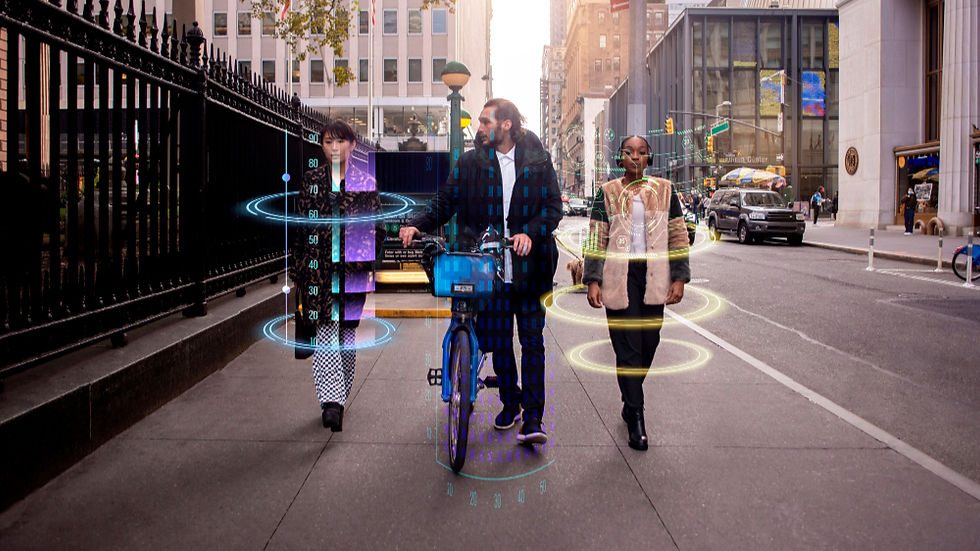
Machine Learning based Motion Detection for Pedestrian Monitoring
Client Spotlight
Our client, a city transportation authority, oversees traffic flow and pedestrian safety in a bustling metropolitan area. With a focus on reducing accident rates and improving infrastructure, they manage various public safety programs. Given the growing challenges posed by increased pedestrian traffic, they sought NEXA's expertise to develop advanced technical solutions for efficient pedestrian monitoring and control.
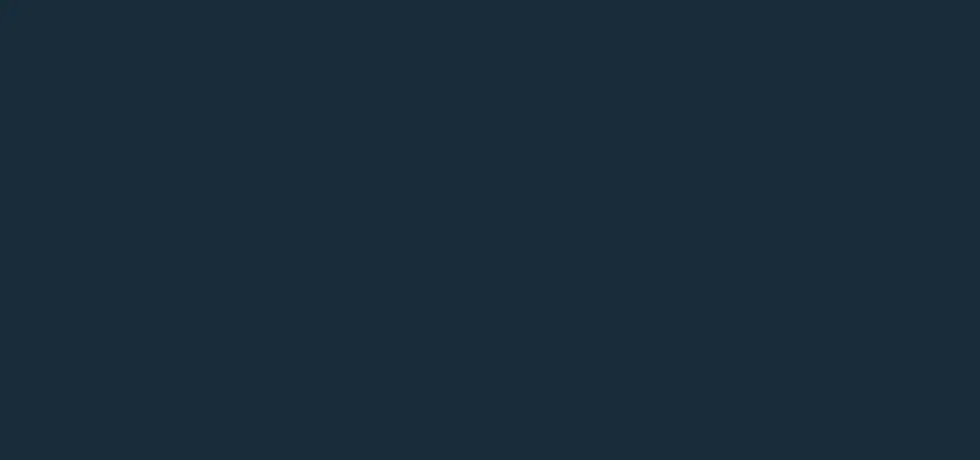.webp)
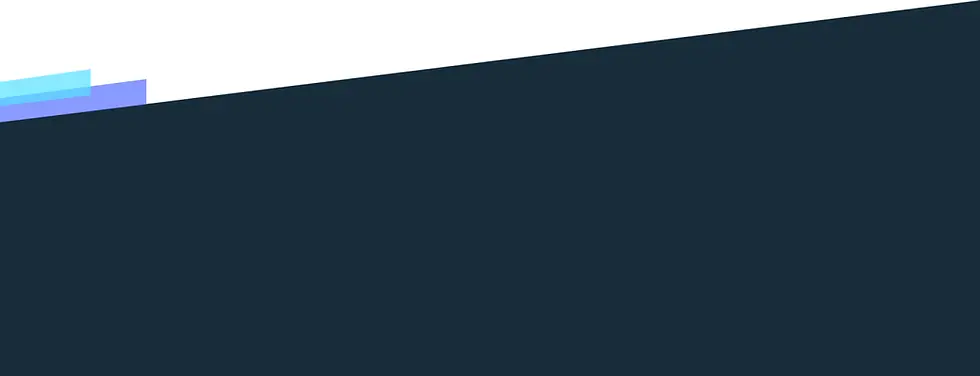.webp)
Results Achieved
Enhanced Monitoring: The machine learning system continuously monitors pedestrian movements, improving the accuracy and efficiency of real-time surveillance.
Actionable Insights: The system generated data-driven insights, such as heat maps of pedestrian traffic, enabling urban planners to redesign traffic patterns and create safer pedestrian zones.
Proactive Safety Measures: The transportation authority implemented targeted safety measures, such as adjusting traffic light timing and improving crossing visibility, leading to a 25% reduction in pedestrian-related accidents in monitored areas within the first year.
User Satisfaction: The system’s reliability and actionable insights were well-received by stakeholders, who praised its role in shifting urban planning toward data-driven decision-making for improved safety and city livability.
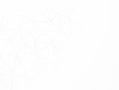
Implementation
Requirements Gathering: NEXA conducted workshops and interviews to understand the client ’s needs and define key performance indicators (KPIs) like peak traffic times and high-risk zones.
System Architecture: A scalable system was designed to integrate seamlessly with the existing surveillance infrastructure, allowing for future expansion and smart city integration.
Machine Learning Model Development: NEXA developed machine learning models using diverse datasets to improve the system's accuracy across different environments and lighting conditions.
Integration and Testing: The system was rigorously tested in real-world environments, fine-tuned based on feedback, and integrated with the city’s camera network to ensure reliable and accurate detection.